Spectral Library Search

PEAKS provides a state-of-the-art library search employing the latest deep learning technology for the identification of peptides from complex data independent analysis (DIA) spectra1. Since DIA spectra contain ions from a precursor mass window, they contain fragment ions from multiple peptides. This results in spectra that are significantly more complex compared to data dependent analysis (DDA) spectra. To accurately identify peptides from these spectra, a spectral library provides a refined search space that matches the fragment ion pattern, expected retention time, and other distinguishing features of the experimental data to previously identified spectra in the library.
With PEAKS all-in-one software, users are able to perform their whole pipeline in one platform, from creating a spectral library to spectral library searching, without sacrificing time and accuracy between converting project results and scoring systems.
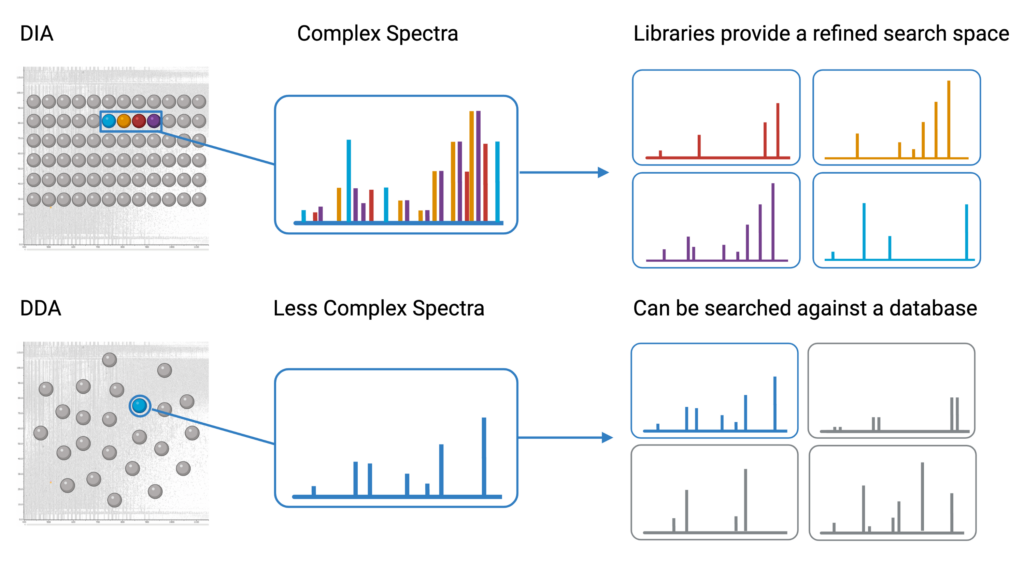
Accurate and sensitive spectral library generation
The first step in the spectral library search workflow is the creation of a spectral library using a DDA mass spectrometry dataset. It is recommended that a fractionated sample is used for library generation in order to obtain deepest proteome coverage and highest number of peptides for the species being studied. Once generated, a spectral library can be used for multiple DIA analyses, and thus its often worth the investment of material and instrument time in developing a comprehensive library. This DDA data is then loaded into PEAKS and searched using the accurate and sensitive PEAKS DB protein database search algorithm. The confidence threshold of the identified peptides can be controlled using the false discovery rate estimated by a target decoy database search. A spectral library can then be generated using the confidently identified peptides.
Each peptide entry in the library will contain useful details from the DDA spectrum that provide the best chance of identifying that peptide within a complex DIA mass spectrometry sample. The precursor mass, ion mobility (if used), and intensity and m/z of the fragment ions that matched the peptide are extracted from the full spectrum. In addition, the retention time (RT) of each library entry is converted to an indexed retention time (iRT). The iRT is generated with an internal library of peptides, it is not necessary to spike in iRT peptides to your library generation and DIA runs. A match between the elution time of the peptide from the library dataset and the experimental dataset can be used to aid the confidence in the spectral library match. If a mass spectrometer is used that can separate peptides based on ion mobility, those details are also included in the spectral library (using the PEAKS IMS add-on module) to improve library search sensitivity.
How to Generate a Spectral Library
Step 1 – Sample preparation: Proteins are digested into peptides, which can then be fractionated
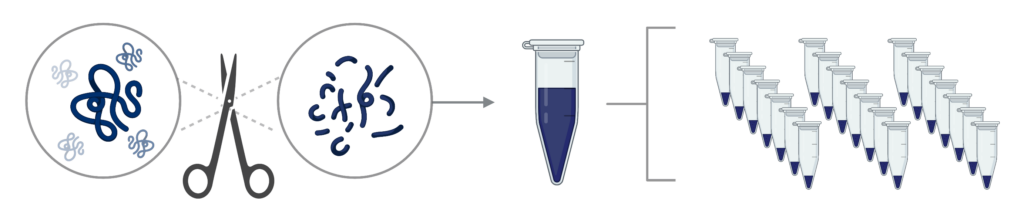
Step 2 – LC-MS/MS: Peptides are analysed using data-dependent analysis (DDA) to generate spectra
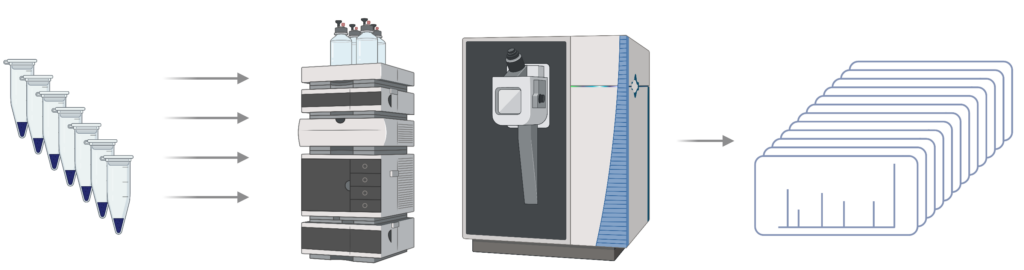
Step 3 – Library generation: DDA data is analysed using PEAKS DB and exported as a spectral library for future analysis of DIA data
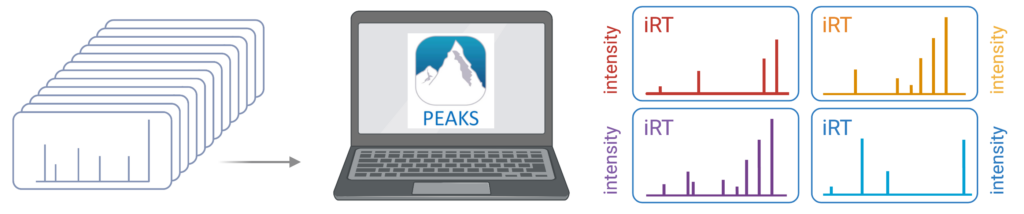
Maximize library match yield with a retention time prediction algorithm powered by deep learning
The library search itself is very easy to use. Spectral libraries can either be setup first before creating a new project or during the library search parameter setup.
When ready to perform the search, add the data of interest to the project, select the library search workflow, and specify the desired spectral library for use with the corresponding search parameters. For large-scale proteomics research projects, PEAKS Online is recommended to not only handle larger cohorts, but also to provide a high-throughput, scalable platform for these comprehensive PEAKS library searches.
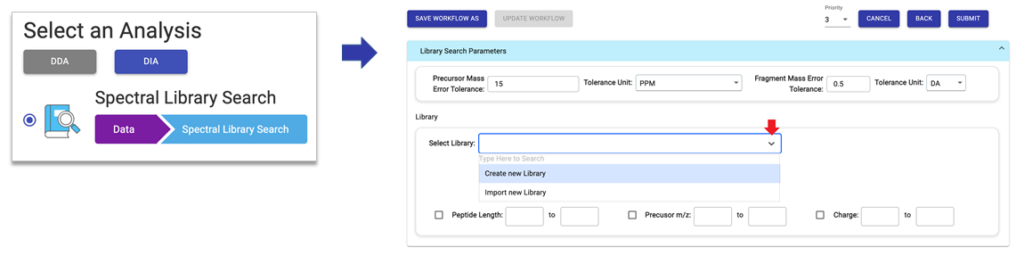
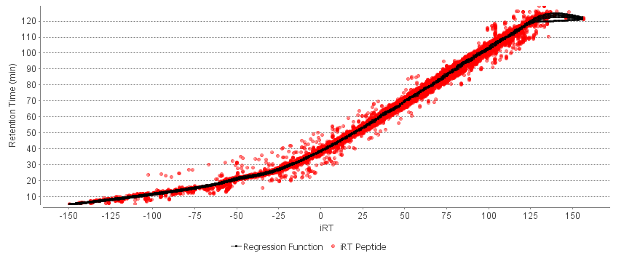
PEAKS uses an advanced peptide feature detection algorithm developed using machine learning to predict the precursor mass and elution profile of the peptides in the sample using the LC-MS information. Details from the spectral library, and a retention time prediction algorithm developed using deep learning are used to predict the elution time of library peptides in the DIA dataset being searched. Peptides from the library are matched to peptide features in the dataset, and the quality of the match is scored based the closeness of fit between the library spectrum and the corresponding MS/MS spectra being searched.
To ensure the accuracy of the results, the false discovery rate (FDR) is predicted using a decoy spectra search (Figure 2). Using the peptides in the spectral library, shuffled peptide sequences are created. Their associated fragment ion lists are also shuffled to create decoy spectra. So, when a spectral library search is run, the software searches a library that contains an equal amount of target spectra and decoy spectra. Based on the spectra that are matched in the result, the false discovery rate can be estimated, and a confidence threshold can be set. For more details about FDR, please see our FDR tutorial.
The result is a list of peptides accurately matched from your DIA spectra to the matching library spectra as evidence of the identifications. Proteins can be inferred from a FASTA file to provide biological context to the identifications. Using the PEAKS’ easy-to-use interface, the library results are graphically presented for easy visual interpretation of the results and comprehensive tables for detailed analysis. The library results can then be carried forward for quantitative analysis. An example of the result view is shown below in Figure 3.
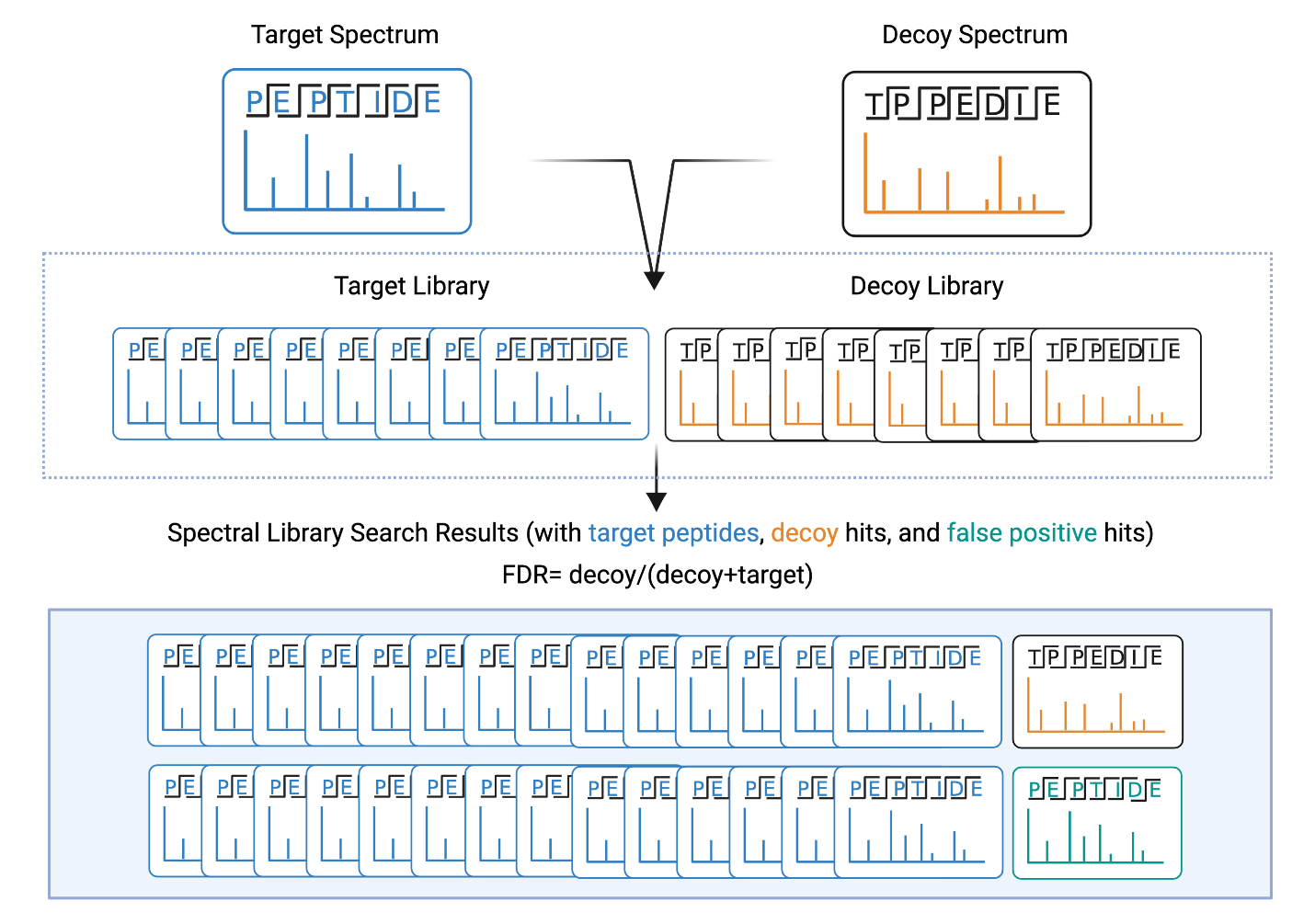
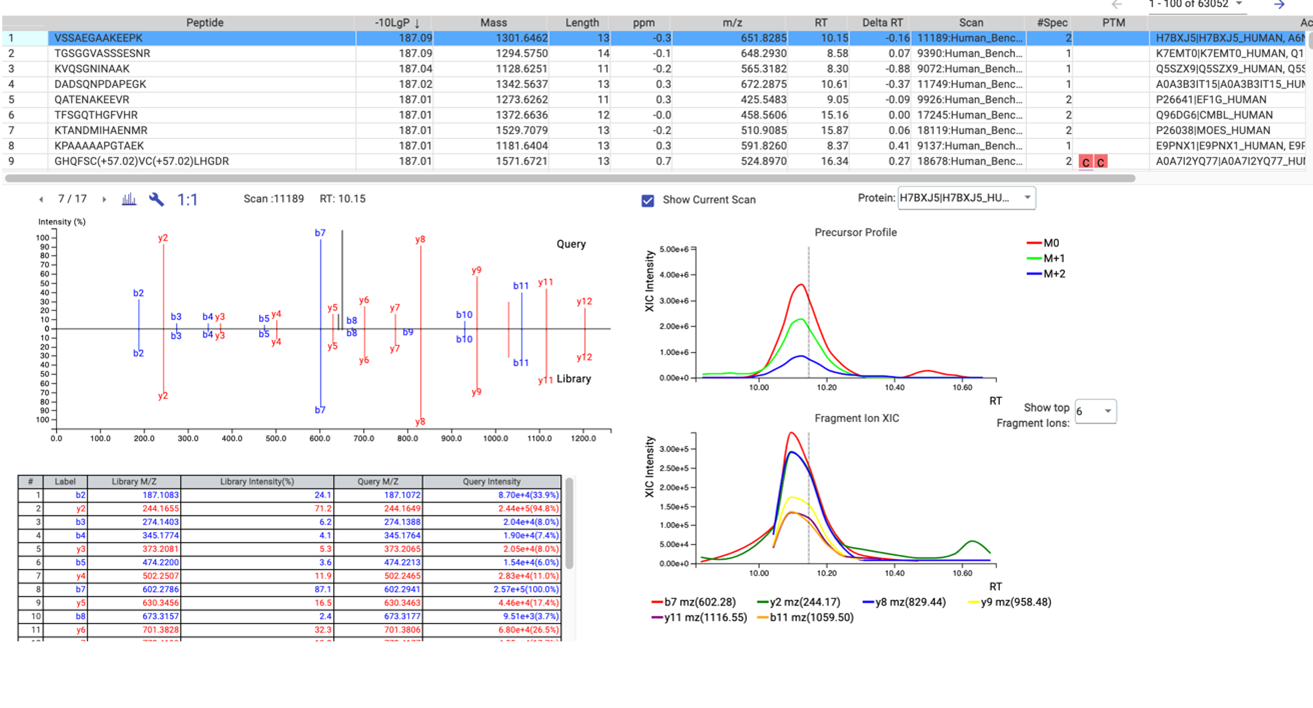
References
- Tran NH, Qiao R, Xin L, Chen X, Liu C, Zhang X, Shan B, Ghodsi A, Li M. Deep learning enables de novo peptide sequencing from data-independent-acquisition mass spectrometry. Nat Methods. 2019 Jan;16(1):63-66. doi: 10.1038/s41592-018-0260-3. Epub 2018 Dec 20. PMID: 30573815.